当前位置:首页 > 过刊浏览->2023年45卷6期
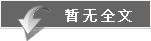
基于机器学习的舰船通信网络故障检测方法
Fault detection method of ship communication network based on machine learning
- DOI:
- 作者:
- 潘志安, 庞国莉, 王小英
PAN Zhi-an, PANG Guo-li, WANG Xiao-ying
- 作者单位:
- 防灾科技学院 信息工程学院, 河北 三河 065201
School of Information Engineering, Institute of Disaster Prevention, Sanhe 065201, China
- 关键词:
- 机器学习;舰船通信;网络故障;稀疏性;神经网络;Softmax回归
machine learning; ship communication; network failure; sparsity; neural network; Softmax regression
- 摘要:
- 为提升舰船通信质量,提出基于机器学习的检测通信网络故障检测方法。在机器学习的自编码神经网络内,添加稀疏性限制与输入数据相关性限制,建立相关性稀疏自编码神经网络;通过逐层贪婪法,预训练神经网络,结合反向传播算法有监督微调神经网络,确定神经网络最佳权值与偏置;在最佳参数确定后的神经网络输入层内,输入原始舰船通信网络数据,利用神经网络隐层提取通信网络故障特征,以Softmax回归分类器为神经网络的输出层,在该层内输入故障特征,以最小错误率为决策准则,输出舰船通信网络故障检测结果。实验证明:该方法可有效提取舰船通信网络故障特征,且各类型故障特征提取结果的边界较为清晰;该方法可精准检测通信网络故障。
In order to improve the quality of ship communication, the fault detection method of detection communication network based on machine learning is studied. In the self-coding neural network of machine learning, the sparse restriction and the correlation restriction of input data are added to establish the correlation sparse self-coding neural network; The optimal weight and bias of the neural network are determined through the layer-by-layer greedy method, the pre-training of the neural network, and the supervised fine tuning of the neural network combined with the back-propagation algorithm; In the input layer of the neural network after the optimal parameters are determined, input the original data of the ship communication network, extract the fault characteristics of the communication network using the hidden layer of the neural network, take the Softmax regression classifier as the output layer of the neural network, input the fault characteristics in this layer, take the minimum error rate as the decision criterion, and output the fault detection results of the ship communication network. The experimental results show that this method can effectively extract the fault features of the ship communication network, and the boundaries of the fault feature extraction results of each type are relatively clear. This method can accurately detect communication network faults.
2023,45(6): 166-169 收稿日期:2022-10-30
DOI:10.3404/j.issn.1672-7649.2023.06.032
分类号:TP391
基金项目:中央高校基本科研业务费专项资金创新团队资助计划项目(ZY20180125)
作者简介:潘志安(1983-),男,硕士,副教授,研究方向为软件定义网络及计算机网络