当前位置:首页 > 过刊浏览->2023年45卷8期
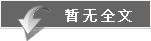
人工智能在船舶航行数学建模中的应用
Application of artificial intelligence in mathematical modeling of ship navigation
- DOI:
- 作者:
- 白灵1, 赵珈玉1, 鞠岩松2
BAI Ling1, ZHAO Jia-yu1, JU Yan-song2
- 作者单位:
- 1. 长春电子科技学院 光电科学学院, 吉林 长春 130000;
2. 长春理工大学 化学与环境工程学院, 吉林 长春 130000
1. College of Optoelectronic Science, Changchun College of Electronic Tecnology, Changchun 130000, China;
2. College of Chemical and Environmental Engineering, Changchun University of Science and Tecnology, Changchun 130000, China
- 关键词:
- 人工智能;船舶航行;数学建模;优化求解;蚁群算法;Dijkstra算法
artificial intelligence; ship navigation; mathematical modeling; optimization solution; ant colony; Dijkstra algorithm
- 摘要:
- 为保障船舶在海上安全航行,提出人工智能在船舶航行数学建模中的应用。使用Maklink图论方法描述海上作业点分布,建立作业点Maklink连接图,生成船舶在作业水域内可航行网络图。建立船舶在海上作业区域航线规划数学模型,并设置约束条件;利用Dijkstra算法求解船舶在海上作业危险区域航线规划模型,得到船舶航行初始航线;利用人工智能算法内的蚁群优化算法对船舶航行初始航线实时优化处理,得到船舶航行最终航线,为船舶穿越海上作业区域实时导航。实验结果表明,该方法可有效生成船舶在作业水域航行网络图,得到初始航线并对初始航线优化处理,应用效果较佳。
In order to ensure the safe navigation of ships at sea, the application of artificial intelligence in mathematical modeling of ship navigation is proposed. Use Maklink graph theory to describe the distribution of offshore construction points, establish Maklink connection diagram of construction points, and generate navigable network diagram of ships in construction waters. Establish a mathematical model for the route planning of ships in the offshore construction area, and set constraints. The Dijkstra algorithm is used to solve the route planning model of ships in dangerous areas of offshore construction, and the initial route of ships is obtained. The ant colony optimization algorithm in the artificial intelligence algorithm is used to optimize the initial route of the ship in real time, and the final route of the ship is obtained, which is used for the real-time navigation of the ship crossing the offshore construction area. The experimental results show that this method can effectively generate the navigable network diagram of the ship in the construction waters, the initial route and the initial route can be better optimized, and the application effect is better.
2023,45(8): 173-176 收稿日期:2022-12-01
DOI:10.3404/j.issn.1672-7649.2023.08.034
分类号:TP391
作者简介:白灵(1991-),女,硕士,讲师,研究方向为应用数学